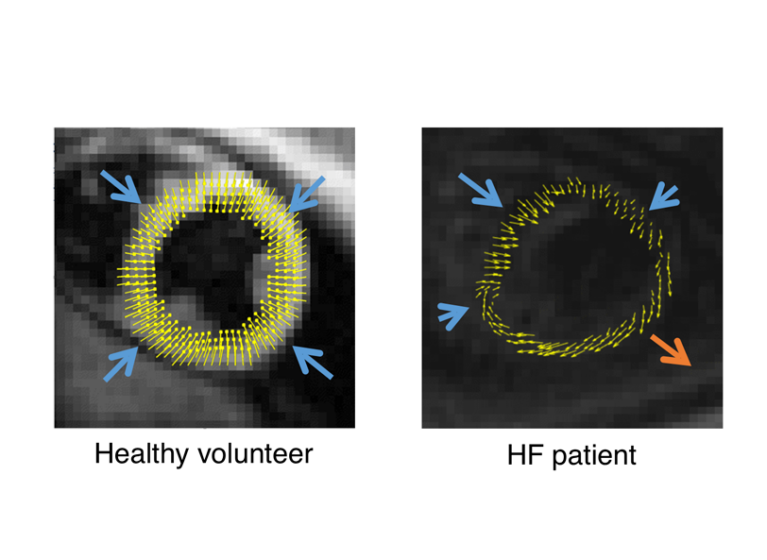
A computer scientist engineer, a biomedical engineer, and a cardiologist are collaborating to develop advanced artificial intelligence (AI) algorithms to improve outcomes for heart failure patients needing cardiac resynchronization therapy (CRT).
Miaomiao Zhang, PhD, is using advanced machine learning to create AI algorithms that analyze magnetic resonance imaging (MRI) studies that show the heart muscle in action.
“One doctor has a limited time capacity and brain power, so we train a machine to look at a gazillion heart images to summarize the best information that can contribute to the clinical decision or diagnosis,” Zhang says.
Maximizing CRT Treatment for More Patients
Heart failure and heart rhythm disorders are common. Patients can develop irregular electrical pathways or scarring in the heart muscle, causing one or more chambers to beat out of rhythm. Some particular devices, like implantable cardioverter-defibrillators (ICDs) and pacemakers, have the capability to deliver CRT.
During CRT, the device sends electrical impulses through electrodes to affected heart muscles, telling them when to fire. Optimal positioning of the electrodes can make a big difference in whether a patient experiences substantial improvement in heart failure symptoms and prognosis from CRT.
Experts at UVA Health use specialized imaging called cine displacement encoding using stimulated echoes MRI (DENSE MRI) to determine whether CRT is likely to benefit a patient and to identify the optimal location for CRT electrode placement. Frederick Epstein, UVA’s Mac Wade Professor of Biomedical Engineering and professor of radiology and medical imaging, and UVA Health cardiologist Kenneth Bilchick, MD, developed DENSE MRI.
Even with these advancements, there are some important knowledge gaps, and the treatment doesn’t always work, Bilchick says.
“In a nutshell, we have a therapy that benefits many patients and can save lives, but some do not respond in the way we would like them to respond,” Bilchick says. “Sometimes that outcome can be improved by just changing the ways we do things.”
In order to analyze UVA Health’s CRT MRI imaging database, Zhang is creating algorithms that train the AI to “mimic how the human brain works.” This could have a big clinical impact, as AI tools can analyze data very quickly and make accurate predictions. When a new patient is being evaluated for CRT, cardiologists can use previously learned data to predict a patient’s outcome, Bilchick says.
Using AI to Automate MRI Analysis
Zhang will use a unique dataset from more than 200 of Bilchick’s patients treated with CRT at UVA Health to train her algorithms. The anonymized patient data includes demographics, other health risks, and cine DENSE MRIs. This imaging is completed before the CRT implant and for several years after to assess outcomes.
The dataset will teach the AI algorithms how to identify abnormalities from standard MRIs. In this way, Zhang hopes to effectively automate MRI analysis.
She was inspired to use AI to develop patient care systems during her postdoctoral training at the Massachusetts Institute of Technology. She noticed how much time neurosurgeons spent studying images during procedures to make treatment decisions.
“We have a 20-year gap in the medical computer technology,” Zhang says. “We could do way better than the software they were using. Machine learning and AI could replace the heavy burden and lots of work.”
Blazing a Trail Toward Clinical Testing
The National Institute of Biomedical Imaging and Bioengineering granted Zhang a Trailblazer R21 Award to further her advanced AI research. She’ll receive $660,000 over 3 years as part of the NIH’s program for exploratory or developmental research.
Zhang’s goals for the first 2 years include:
- Completing development of AI algorithms using the UVA Health cine DENSE MRI dataset
- Using all image modalities to teach the AI to detect heart anomalies such as arrhythmias or scar tissue
- Training the AI to pinpoint the best location for pacemaker or defibrillator lead implants
In the final year of the grant, Zhang hopes to deliver software that Bilchick and others can use for clinical testing.
“We will continually refine these algorithms to get the best performance,” Zhang says. “We hope through this project that we can somewhat automate the entire process of analyzing images and providing the right information in a clinical setting.”
Eventually, Zhang wants to generalize her AI technology so other clinical centers can use it for multidisciplinary studies.
Expanding Options for Personalized Care
Zhang’s methodology goes beyond a traditional formula with known inputs, Bilchick says.
“This is about using technology to work like a human brain and see overall patterns, but with features you might not have identified from the start,” he says. “Down the road, AI algorithms like the ones we are using now could be used with more complex and newer technologies.”
This ability to train AI to complete “deep learning” opens the potential for more personalized care for CRT patients. Clinicians no longer need to rely on prescribing medical therapy with a one-size-fits-all concept. AI can:
- Factor in differences among patients
- Identify the best approach for a specific patient with a unique set of symptoms
For Zhang, success means creating a fast, accurate, and automatic process for evaluating MRI images that can be used at many clinical centers, including those without a DENSE MRI facility. This would be a major equalizer, enabling clinicians to improve CRT care worldwide, Zhang says.