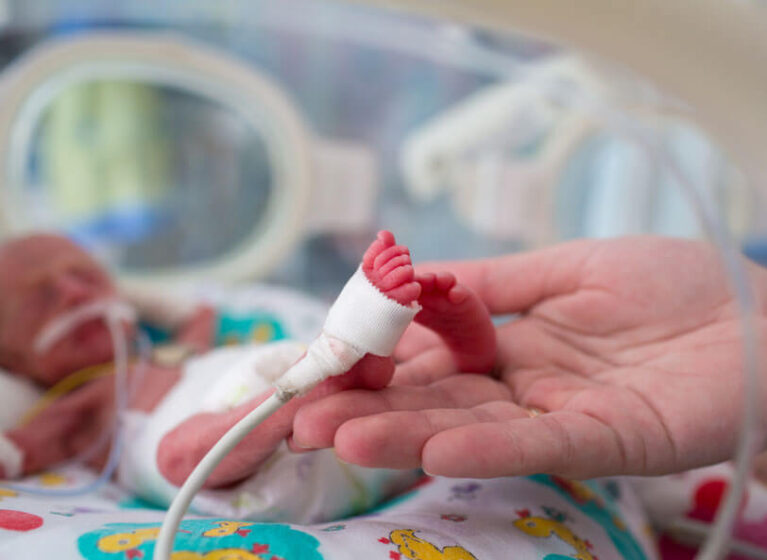
In the neonatal intensive care unit (NICU), continuous monitoring alerts doctors to vital sign changes. Heart rate, respiratory rate, blood pressure, and oxygen saturation offer insight into an infant’s condition. But even with all these data points and an experienced team, a baby’s condition can deteriorate rapidly.
Conditions like sepsis and necrotizing enterocolitis (NEC) cause significant morbidity and mortality in premature neonates. Another condition, neonatal opioid withdrawal syndrome (NOWS) also affects neonates born at term. These conditions are preceded by subtle vital sign changes that are often missed by conventional monitoring.
Predictive analytics uses machine learning and AI to pick up these subtle changes. This means recognizing illness sooner and more reliably. Early detection can make the difference between life and death.
Predictive Analytics Offers Multiple Applications
At UVA Health Children’s, neonatologists Brynne Sullivan, MD, and Karen Fairchild, MD, lead multicenter studies on using predictive analytics to improve NICU outcomes.
Powered by the world’s largest collection of NICU vital sign data, UVA Health Children’s and the UVA Center for Advanced Medical Analytics (CAMA) explore the ways that predictive analytics improves care. While the potential applications are innumerable, the researchers are specifically looking at sepsis, NEC, and NOWS.
POWS for Opioid Withdrawal
A troubling rise in opioid use and addiction nationwide also means more newborns are affected. NOWS cases have been increasing at a rate that mirrors the increased opioid abuse rates. Because the symptoms of NOWS are variable and non-specific, there are often treatment delays with lifelong consequences for the child.
However, opioid withdrawal affects the autonomic nervous system, which means it can be analyzed through vital signs. A novel system aims to use a pulse oximeter withdrawal score (POWS) to assess symptoms and guide treatment.
UVA Health Children’s and CAMA have an additional asset for monitoring potential opioid withdrawal. They capture bedside monitoring data from all of UVA Health’s intensive care units.
Preventing Sepsis & NEC Deterioration
Sepsis and NEC both show preceding patterns in the vital signs of patients before symptoms begin. “Sepsis and other conditions can cause similar patterns,” Sullivan says. “But the end result is deterioration which requires increased support, and often derails a baby’s healthy trajectory.”
Conventional vitals reporting only presents a snapshot in time. These fleeting values don’t capture trends or patterns. Even the most sophisticated monitors cannot detect the patterns identified by advanced time-series analytics, machine learning, and AI.
These analytics allow for precision medicine -- treatment that’s focused and timely. Building these tools requires data. A lot of it.
Big Answers Require Big Data
These vital signs eventually become data points in expansive datasets. The datasets are designed to be shareable as discoveries are made and solutions created. As data scientists, data engineers, and clinicians work together to assemble pieces of each patient’s story, cooperation is vital. “Big data analytics is a key aspect of our research,” says Fairchild.
Another important collaboration is with other NICUs. While UVA Health Children’s treats over 600 infants per year in our NICU, multicenter datasets test the generalizability of models and results. Combining data sets from partnerships formed with other NICUs around the country creates a more complete picture of how these conditions emerge and what course they generally follow. Fairchild says, “Collaborations are key — you cannot thrive or survive without them. You cannot survive in the competitive world of biomedical research if you work in a silo.”
But the biggest question is how clinical teams will be able to use this data. “We want to integrate this into the clinical workflow in a way that the medical team will benefit from using it and wants to use it,” Sullivan says.
Fortunately, we already have a model that shows how predictive analytics can improve outcomes.
Giving Doctors New Ways to be a HeRO
In the early 2000s at UVA Health, cardiologist Randall Moorman, MD, pioneered the well-known HeRO system. HeRO uses heart rate changes to predict sepsis in preterm babies. By alerting doctors when a baby’s heart rate characteristics fit the model seen in other sepsis patients, doctors have more time to act. The largest clinical study looking at HeRO showed it reduced sepsis-associated mortality by 40%.
But heart rate isn’t the only way to predict illness. “When babies in the NICU get sick they often present with a change in breathing,” Sullivan says. New analytic tools, like the pulse oximetry warning system, use heart rate and respiratory patterns as predictors.
Like HeRO, the analytic tools being developed:
- Track changes in vital signs
- Generate a risk score
- Display risk score trends on a monitor
- Create alerts as needed
While AI and machine learning can digest data more quickly than a human, once the score is generated, clinical experience is crucial. Human intelligence and experience determine whether to treat or watch and wait.
One Solution Addresses Many Problems
Predictive analytics has established success. The HeRO system reduced sepsis-related mortality in a large, multicenter randomized clinical trial in premature infants. Its impact in this trial shows that doctors use the displayed information to alter care and improve outcomes.
As more datasets are collected and patterns emerge, predictive analytics can address not just sepsis, but other causes of neonatal morbidity and mortality, like NEC and severe opioid withdrawal. With multidisciplinary and multicenter collaboration, expansive datasets can be compiled to fuel the learning models and create better systems for early detection.